Events
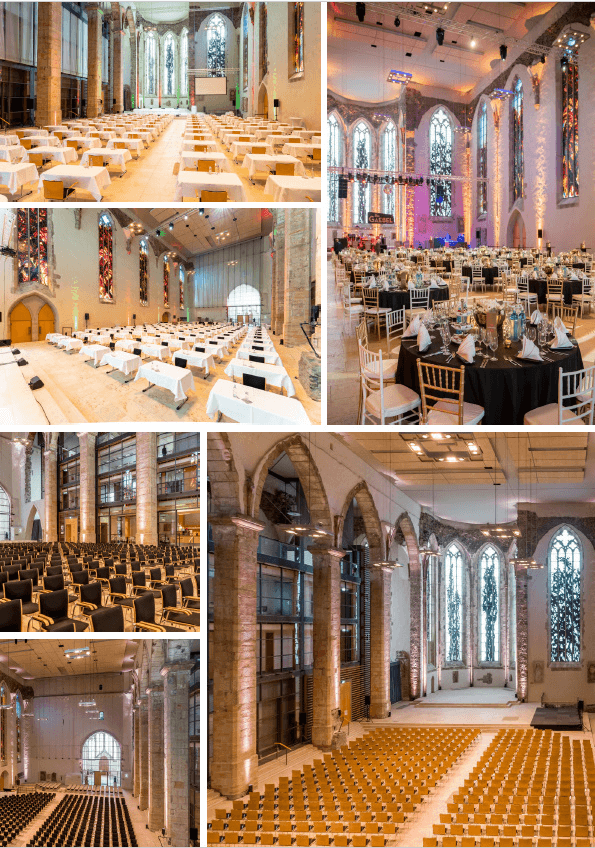
Top Event
16.-18., Jun, 2025.
2nd International Workshop on Reacting Particle-Gas Systems, Mageburg, Johanneskirche
We are pleased to announce the 2nd International Workshop on Reacting Particle-Gas Systems organized by the Collaborative Research Centre 287 BULK-REACTION. The workshop will take place in a very special event location, the Johannis-Kirche in Magdeburg, Germany. The core topic of the workshop are going to be reacting particle systems and scientific work dealing with experiments, simulations or measuring techniques in these type of systems will be presented. An official call for abstracts will follow soon. Selected contributions will also be featured in a special issue of a peer-reviewed journal.
(Workshop)
26., Sep, 2024.
1st doctoral researcher’s self-organized workshop in funding period 2
The first doctoral researcher’s self-organized workshop in funding period 2 took place in Bochum in September 2024. It included a data-sprint to foster RDM best practices. There was a guest lecture by Anika Weber (PhD student at DLR) on the topic of Development, Modelling, and Experimental Investigation of a New, Innovative Particle Concept for Solar Fuel Production.
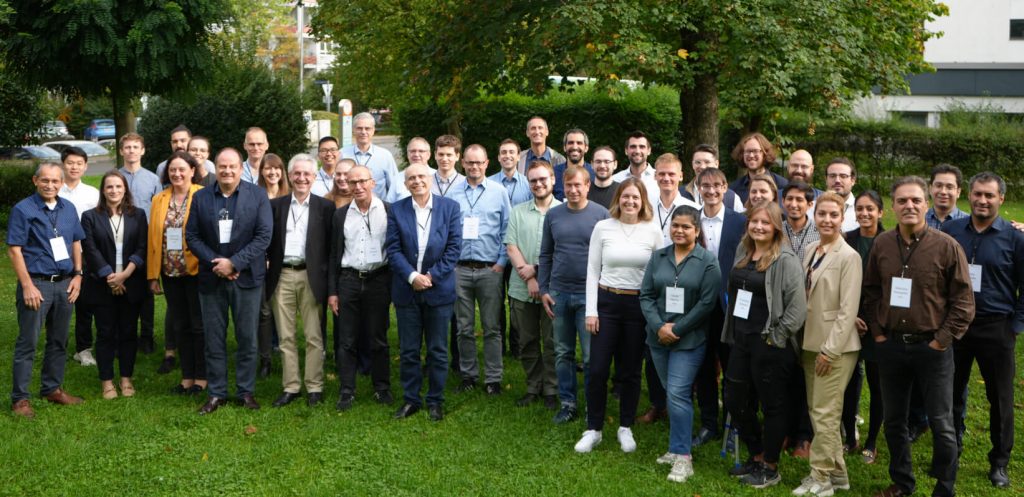
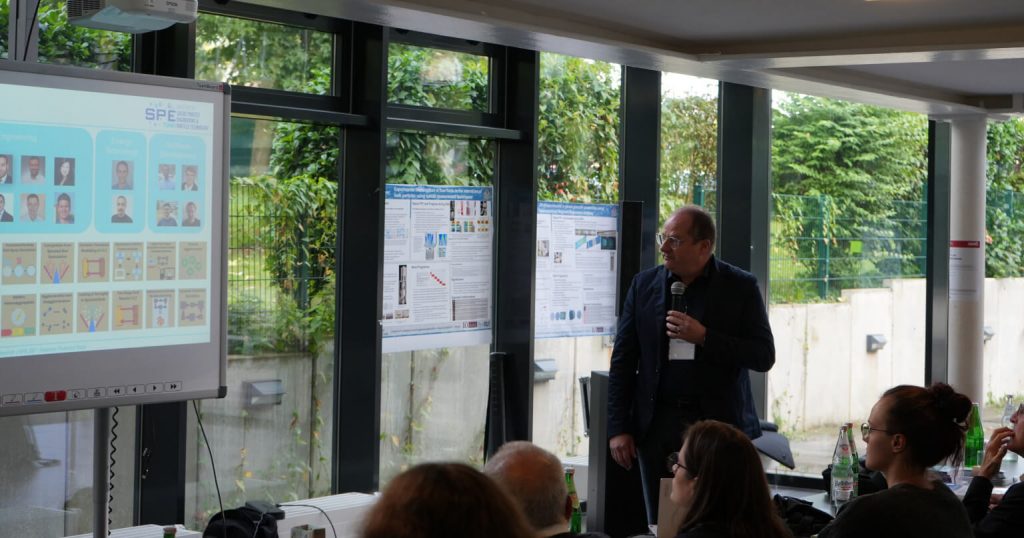
24.- 25., Sep, 2024.
3rd Retreat of the CRC/TRR 287 BULK-REACTION in Bochum
The 3rd Retreat of the CRC/TRR 287 BULK-REACTION took place in Bochum in September 2024. It was the first in-person meeting of all CRC members after the 2nd funding period was granted by the DFG in may this year. The team discussed the current status and the strategic orientation of the CRC and a group of new PhD students was welcomed. Prof. Stefan Heinrich (TU Hamburg), invited guest speaker, held a key note lecture about reactive fluidized beds, presenting state-of-the-art measurements techniques in the field of particle technology and giving insight into a topic that is of great interest to the CRC.
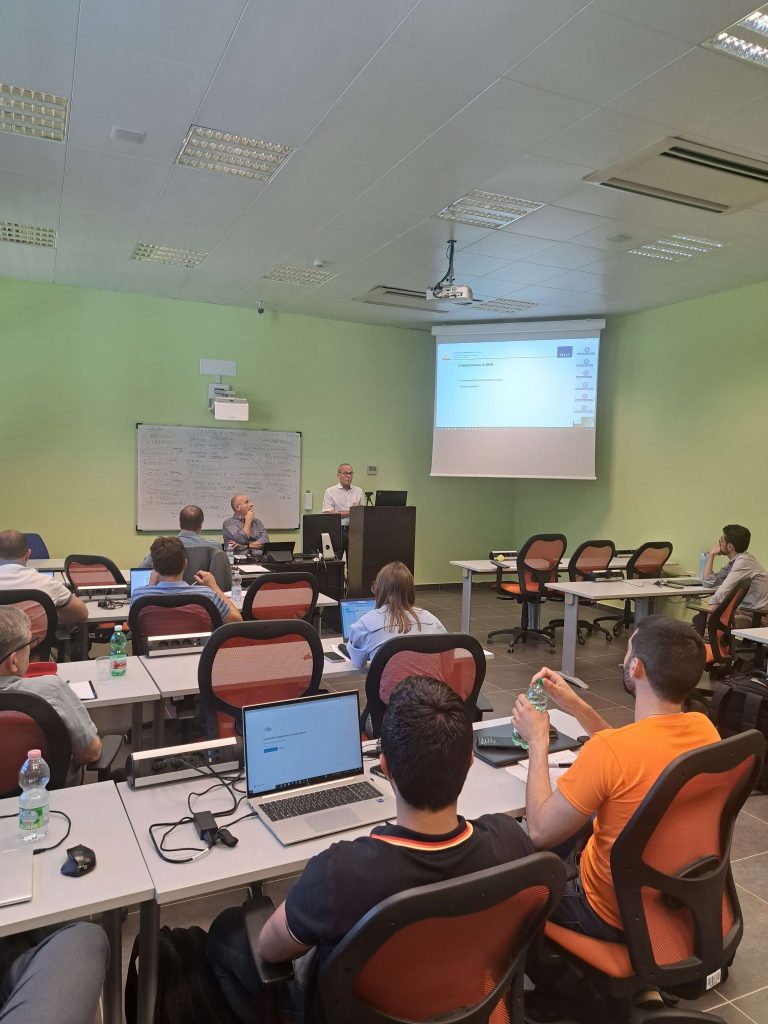
13., June, 2024.
Prof. Scherer gave a seminar at University of Salerno, Italy, on June 13 2024 on “DEM/CFD simulation of moving and reacting granular assemblies with gas flow”
(IPNF 2024)
11.- 12., June, 2024.
Prof. Scherer was a keynote speaker at the Modeling and Simulation Session during the International Powder & Nanotechnology Forum (IPNF 2024) in Frankfurt on June 11-12, 2024, entitled “DEM/CFD simulation of chemically reacting, thermally thick particles in moving granular assemblies with gas flow: challenges and recent developments.”
See website for IPNF 2023:https://ipnf2023.com/. The website for IPNF 2024 is yet not online but to be published soon.
Conference
7.- 10., May, 2024.
The CRC 287 organized a mini-symposium during the forthcoming International Conference on Numerical Combustion, Kyoto, Japan (7-10 May 2024) on the topic of tabulation approaches for homogeneous and heterogeneous reacting flows.
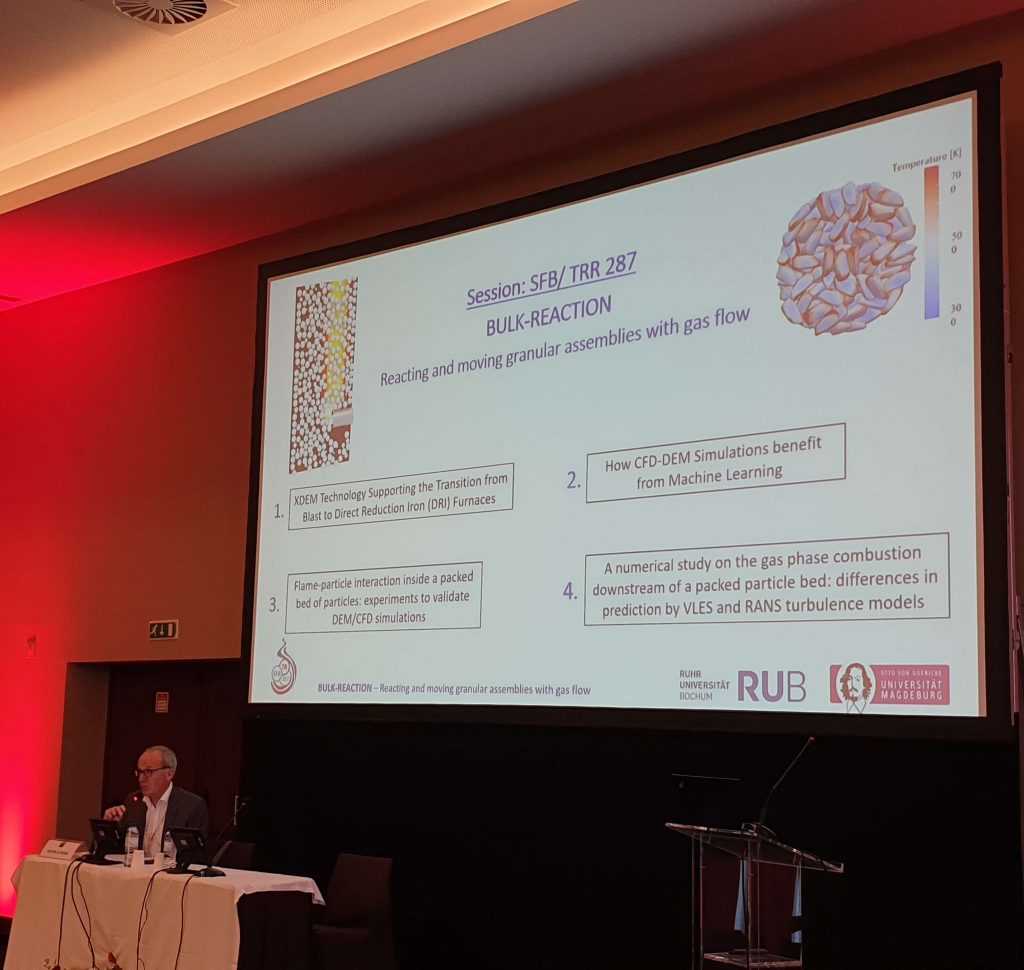
2.- 5., April, 2024.
The CRC 287 organized a dedicated session at the upcoming Conference on Industrial Furnace and Boilers (2-5. April 2024, Portugal, Algarve)
This session includes invited lectures of Prof. Bernhard Peters, Luxembourg (XDEM technologies supporting the transition from blast to direct reduction of iron furnaces) and Prof. Stefan Radl (How CFD-DEM Simulations benefit from machine learning), Graz University of technology. These lectures were complemented by contribution of the CRC 287 (measurements on flame wall interaction in packed beds that allows for the validation of DEM/CFD, assessment of the differences of RANS and VLES simulations on the flame structure downstream of packed beds)
Soft skills lecture
Soft skills lecture
18, Dec., 2023, 11:00-13:00
Bookreading: Parenthood and science – (in)compatibilities, Corona Gaps and us.
During this lunch lecture, Dr. Sarah Czerney and Dr. Lena Eckert gave an insight into their books.
Sarah Czerney, Lena Eckert, Silke Martin (2022) Mutterschaft und Wissenschaft in der Pandemie: (Un-)Vereinbarkeit zwischen Kindern, Care und Krise, Verlag Barabara-Budrich-Verlag, ISBN: 978-3-8474-2654-7.
Sarah Czerney, Lena Eckert, Silke Martin (2020) Mutterschaft und Wissenschaft: Die (Un-) Vereinbarkeit von Mutterbild und wissenschaftlicher Tätigkeit, Springer Verlag, ISBN: 978-3658309312.
In order to explore the tension between parenthood, motherhood, other unpaid care work and science. After the lecture, participants had the opportunity for discussion.
Further reading:
Sarah Czerney, Lena Eckert, Silke Martin (Hg.): Mutterschaft und Wissenschaft in der Pandemie. (Un-)Vereinbarkeit zwischen Kindern, Care und Krise. Budrich: 2022
“Nähkästchen extended: Netzwerk Mutterschaft und Wissenschaft” – Podcast from University of Hamburg
“KARRIERE NUR OHNE KINDER?” – Interview with rbb kultur
“Ungleichheit bei Unikarrieren: Forscherinnen durch Pandemie benachteiligt” – Report in Tagesspiegel
Speaker
Dr. Sarah Czerney and Dr. Lena Eckert (Netzwerk Mutterschaft und Wissenschaft)
MGK Guest lecture
MGK Guest lecture
14, Dec., 2023 at 3:00-4.30 PM
MGK Guest lecture by Prof. Vikranth Kumar Surasani.
Title: “Investigation on CO2 geological sequestration in the deccan volcanic province through multiphase flow and reactive transport simulations”
The seminar took place online via Zoom.
Industrial activity is the primary source of carbon emissions with a estimated 78% of total contribution. Carbon capture and sequestration (CCS) technology is one of the alternatives to reduce CO2 emissions, where the captured CO2 is transported to the facilities using various storage techniques. Geologic carbon sequestration (GCS) is one such technique, where deep geological subsurface formation is used as permanent storage facility. Based on available geological, petrophysical, and geochemical properties, the Deccan volcanic province is one of India’s largest sinks for geological CO2 sequestration. Approximately 5,000,000 km2 of India is covered with Deccan basalt. The fate of injected CO2 in the geological formations follow various physical trappings and chemical transformations which are classified as structural, residual, solubility, and mineral trapping mechanisms.
The current research investigates the possible implementation of CO2 geological sequestration in the Deccan volcanic province. The numerical simulation analysis is carried out to analyse the influences of specific sequestration parameters like the injection rate and injection point; and geological parameters like top surface morphology, petrophysical properties variation, and geological features like stairsteps traps and anticline on the CO2 plume migration in the subsurface formation domain. The effect of these sequestration and geological parameter variations is analysed on the trapping mechanism, sweeping efficiency and structural integrity over a geological time scale for the considered subsurface synthetic domain. The multiphase, multicomponent reactive transport modelling technique is utilized to conduct this analysis. The outcome of this research has shown insight into the potential implementation of CCS and a future estimate of CO2 migration and CO2 entrapment. Further, provide the impacts of sequestration and geological parameters over a geological time scale. Future studies will focus on caprock leakage analysis, leakage implications on different entrapment mechanisms and machine learning approaches to predict the entrapment percentage, leakage, and structural integrity research for long geological time scales.
Keywords: Carbon Capture and Storage, Geologic Carbon Sequestration, Trapping mechanisms, Sequestration parameters, Geological parameters, Reactive transport modelling.
Soft skills workshop
Soft skills workshop
13, Dec., 2023, 10:00-12:00
Workshop on “Gender appropriate candidate assessment” in Magdeburg by Dr. Steffi Nothnagel (Diversity Consulting)
The workshop addressed gender appropriate candidate assessment during recruitment processes. It presented strategies and good-practice examples on how to avoid gender bias within the recruiting process based on equal treatment of all applicants. Established approaches at both universities were discussed and evaluated with regard to opportunities for improvement.
Lecture
Lecture
4-6, Oct., 2023
Lecture on “Modelling the combustion/gasification of biomass in fixed beds: an Eulerian-Eulerian approach” in Bochum and Magdeburg by Prof. Porteiro.
Prof. Porteiro is Director of Sustainability Area at the Unviersity of Vigo (Spain) and an Expert in CFD simulations, combustion and biomass conversion processes. He visited Bochum and Magdeburg and gave a presentation at both locations. The presentations are in presence and the topic of the presentation is “Modelling the combustion/gasification of biomass in fixed beds: an Eulerian-Eulerian approach“.
1. Presentation
Bochum, Wednesday, 4th of October
Time: 11:00 – 12:00
Location: Room IC2 156/90
2. Presentation
Magdeburg, Friday, 6th of October
Time: 10:00 – 11:00
Location: Building 10, Room 219
MGK Guest lecture
MGK Guest lecture
20, Sep., 2023 at 10
MGK Guest lecture by Prof. Bénédicte Cuenot, CERFACS, France.
Title: DNS and LES of turbulent reacting gas flows with detailed kinetics and liquid or solid particles
MGK Guest lecture
MGK Guest lecture
16, Aug., 2023 at 10:00 am
MGK Guest lecture by Dr. Rigopoulos, Imperial College London.
Title: “Machine Learning for Thermochemistry Tabulation”
The seminar will take place in lecture theatre H2 (room number A021) on the ground floor of Building 22A in Magdeburg. You may also participate electronically.
Several methods for the computation of reacting flows involve real-time integration of chemical kinetics. These methods include transported probability density function (PDF) methods, direct numerical simulation (DNS), conditional moment closure (CMC), unsteady flamelet, multiple mapping closure (MMC), thickened flame model, linear eddy model (LEM), partially stirred reactor (PaSR, as in OpenFOAM) and laminar flame computation. The need for direct integration of kinetics often renders large-scale simulations with these methods prohibitively expensive. This issue can be overcome by tabulation, which is the pre-computing and storage of computationally expensive operations. Tabulation raises further issues, however, such as the anticipation and coverage of the states to be encountered during simulation and the avoidance of excessive memory requirements.
The present seminar described a tabulation approach with artificial neural networks (ANNs), which are a class of machine learning models that can be employed for function approximation. After a review of the first-generation ANN tabulation methods, we discussed recent developments that enable the generation of data-driven models able of accommodating a wide range of reacting flows, including laminar flamelets and premixed flames, turbulent non-premixed and premixed flames and flames with fuel blends and energy losses. The main elements of the methodology were discussed, together with an explanation of how they lead to enhanced generalisation, accuracy and error control.
April 24th – 26h, 2023
Rouen, France
August 30th – September 2nd, 2022
Budapest, Hungary
June 8th – 9th, 2022
Ruhr-University Bochum, Germany
organized by the Collaborative Research Centre 287 BULK-REACTION